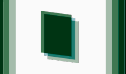
ABSTRACT
There is an increasing interest in image-to-image translation with
applications ranging from generating maps from satellite images to
creating entire clothes’ images from only contours. In the present
work, we investigate image-to-image translation using Generative
Adversarial Networks (GANs) for generating new data, taking as a
case study the morphing of giraffes images into bird images. Morphing
a giraffe into a bird is a challenging task, as they have different
scales, textures, and morphology. An unsupervised cross-domain
translator entitled InstaGAN was trained on giraffes and birds,
along with their respective masks, to learn translation between
both domains. A dataset of synthetic bird images was generated
using translation from originally giraffe images while preserving
the original spatial arrangement and background. It is important to
stress that the generated birds do not exist, being only the result of a
latent representation learned by InstaGAN. Two subsets of common
literature datasets were used for training the GAN and generating
the translated images: COCO and Caltech-UCSD Birds 200-2011.
To evaluate the realness and quality of the generated images and
masks, qualitative and quantitative analyses were made. For the
quantitative analysis, a pre-trained Mask R-CNN was used for the
detection and segmentation of birds on Pascal VOC, Caltech-UCSD
Birds 200-2011, and our new dataset entitled FakeSet. The generated
dataset achieved detection and segmentation results close to
the real datasets, suggesting that the generated images are realistic
enough to be detected and segmented by a state-of-the-art deep
neural network.
O Computer on the Beach é um evento técnico-científico que visa reunir profissionais, pesquisadores e acadêmicos da área de Computação, a fim de discutir as tendências de pesquisa e mercado da computação em suas mais diversas áreas.