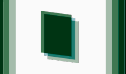
The small and medium-size cities are facing problems related to mobility
that could be avoided by adopting the public transportation
system, as buses and trains. However, in many Brazilian cities the
use of public transportation is neglected because it is considered
uncomfortable, expensive and insecure. To attract passengers for
such kind of transportation there are several possible approaches,
the promotion of comfort perception is one of those. Several studies
have already approached this problem, however, few of them
addressed the perception of comfort felt by the passengers using
telemetry data collected from the vehicle. Among the works that
use such data, none of them applied data mining techniques to
abstract a general model of comfort perception. Therefore, this
work aims to apply mining techniques over telemetry data collected
from vehicles to build a comprehensible model to classify
the level of comfort of public transportation passengers. To achieve
this objective machine learning techniques were used, centered on
decision trees. Due to the complexity of abstracting the model there
were constructed three models, one for each acceleration axis that
were merged using a meta-classifier responsible to point out the
passenger general comfort. The results have reached an accuracy
of 85,2%, which can be considered a promising result regarding
the difficulties of separating the data source in sets that can better
identify the bus drivers behaviour.
O Computer on the Beach é um evento técnico-científico que visa reunir profissionais, pesquisadores e acadêmicos da área de Computação, a fim de discutir as tendências de pesquisa e mercado da computação em suas mais diversas áreas.