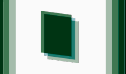
ABSTRACT
In recent years, lung ultrasound (LUS) has gained much importance
in both the clinical and technological environments. This is because
over the years it has been noticed that the presence of artifacts in
the images correlates with a series of anomalies or diseases that
affect the lungs. Therefore, assisting in the segmentation of these
artifacts is of great clinical interest, as the prior identification of any
anomaly can prevent its aggravation. Another challenging scenario,
in particular, is that of emergency units, where fast and accurate
diagnosis is essential, therefore, developing methods that facilitate
the task of identifying anomalies through the segmentation of artifacts
in images is of great importance. In this work, we train and
compare the performance of three deep neural network architectures
that can aid in clinical diagnosis by segmenting B-line artifacts
in LUS images. These trained models are based on semantic segmentation,
in which they perform semantic labeling at the pixel level.
The architectures provided by DeepLabV3+ were used, they were:
Resnet-18, Resnet-50 and Exception. These trained models were
evaluated using the metric IoU (intersection over union), precision,
accuracy, and sensitivity. In the end, the model that proved to be
the most accurate was the one based on the Resnet-18 architecture,
with an accuracy of 92.32%. Resnet-50 also showed satisfactory
results, with a precision of 96.07% and a sensitivity of 91.39%.
O Computer on the Beach é um evento técnico-científico que visa reunir profissionais, pesquisadores e acadêmicos da área de Computação, a fim de discutir as tendências de pesquisa e mercado da computação em suas mais diversas áreas.