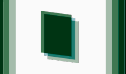
ABSTRACT
Product retrieval from images has multiple applications ranging
from providing information and recommentations for customers
in supermarkets to automatic invoice generation in smart stores.
However, this task present important challenges such as the large
number of products, the scarcity of images of items, differences
between real and iconic images of the products, and the constant
changes in the portfolio due to the addition or removal of products.
Hence, this work investigates ways of generating vector representations
of images using deep neural networks such that these
representations can be used for product retrieval even in face of
these challenges. Experimental analysis evaluated the effect that
network architecture, data augmentation techniques and objective
functions used during training have on representation quality. The
best configuration was achieved by fine-tuning a VGG-16 model
in the task of classifying products using a mix of Randaugment
and Augmix data augmentations and a hierarchical triplet loss as a
regularization function. The representations built using this model
led to a top-1 accuracy of 80,38% and top-5 accuracy of 92.62% in
the Grocery Products dataset.
O Computer on the Beach é um evento técnico-científico que visa reunir profissionais, pesquisadores e acadêmicos da área de Computação, a fim de discutir as tendências de pesquisa e mercado da computação em suas mais diversas áreas.