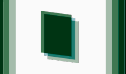
Abstract
The Deep Neural Network architectures’ advances offer innovative
and effective solutions to various complex challenges. In order to
improve the models’ effectiveness based on these architectures’ requirements,
the model hyperparameter optimization is an essential
project stage. Despite this, the existing optimization techniques
demand a high computational cost when directly applied to the
state-of-the-art most complex architectures. In this sense, this study
proposes a method for hyperparameter optimization leveraging
low computational cost for Artificial Intelligence models through
structured pruning techniques. For the purpose of verifying and
demonstrating the method, three main hypotheses are investigated
throughout the implementation of a case study, which consists of
the models’ fine-tuning and optimization for three-dimensional
geometric shapes classification on real-world object images. The
process includes hyperparameter optimization for pruned models,
considering the posterior retraining, evaluation, analysis and comparison
between the performance and efficiency of the original
models. Finally, the results were promising, indicating an improvement
that reaches up to 10.58% Precision by just focusing on the
models’ learning rates optimization.
O Computer on the Beach é um evento técnico-científico que visa reunir profissionais, pesquisadores e acadêmicos da área de Computação, a fim de discutir as tendências de pesquisa e mercado da computação em suas mais diversas áreas.