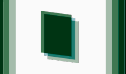
ABSTRACT
Image classification entails assigning labels to an image based on
its visual representation. Among the approaches used for this problem,
the use of neural networks is particularly notable. However,
these algorithms can be hampered by noisy data. Preprocessing
techniques, such autoencoder neural networks, are employed to
mitigate this impact. Therefore, the problem of classifying noisy images
is approached using two models: one for classification and the
other for noise removal. The research question asks about the feasibility
of incorporating information from the classification model
into the development and adaptation of the noise removal model.
The general objective is to explore the possibility on accuracy improving
of an image classifier using an autoencoder for noise removal,
adapted by introducing the classification error metric into
its learning algorithm. The scope of this research is limited to the
challenging problem of classifying handwritten digits in images.
The adopted method is an experimental research consisting of four
stages: (I) evaluation of the classifier’s performance in noise-free
images; (II) analysis of classifier performance in noisy images; (III)
investigation of the impact on classification performance after image
pre-processing; (IV) exploration of the adaptation of the noise
removal model and analysis of its impact on classification. It stands
out as a significant result that the proposed adaptation can improve
the rate of correct classifications for pre-processed noisy images,
in addition to showing signs of making the development of noise
removal models more efficient.
O Computer on the Beach é um evento técnico-científico que visa reunir profissionais, pesquisadores e acadêmicos da área de Computação, a fim de discutir as tendências de pesquisa e mercado da computação em suas mais diversas áreas.