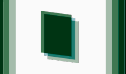
ABSTRACT
Society grapples with an overwhelming and ceaseless flow of information,
posing challenges to a media environment already afflicted
by eroding trust in news. The use of Supervised Learning models
for Fake News classification is widespread, yet their effectiveness
hinges on the quality of labeled data. Constructing datasets that
encompass the intricate nuances of disinformation across diverse
contexts remains a formidable task. This study presents a comparative
analysis of various Supervised Learning models for detecting
and classifying misinformation. Leveraging the LIAR dataset, which
employs six different classes to characterize the veracity of statements,
our findings align with the accuracy benchmarks established
by the LIAR authors. Specifically, the logistic regression model with
stemming achieves an accuracy of 25%. The study suggests potential
enhancements through the application of Deep Learning
techniques, revealing a positive correlation between accuracy and
the number of training epochs. Despite current accuracy levels,
notably lower than datasets with binary classifiers, it is crucial
to underscore the meticulous manual verification and annotation
process executed by the LIAR dataset authors.
O Computer on the Beach é um evento técnico-científico que visa reunir profissionais, pesquisadores e acadêmicos da área de Computação, a fim de discutir as tendências de pesquisa e mercado da computação em suas mais diversas áreas.