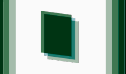
ABSTRACT
Precision agriculture has emerged as a transformative approach
to optimizing crop production and resource management in the
agricultural sector. It leverages advanced technologies like remote
sensing, geographic information systems, and decision support
tools. In recent years, machine learning has become an integral
component of precision agriculture, providing the ability to analyze
large volumes of complex data and generate valuable insights for
more informed decision-making. This study benchmarked different
supervised algorithms in an image classification dataset of coffee
and non-coffee areas. Algorithms were comprehensively evaluated
and analyzed, with traditional machine learning models trained
in three versions of the original dataset, and compared with two
Deep Learning algorithms. The experimental results were promising,
showing that SVMs and RF algorithms can provide accurate
predictions for most images with average accuracy values above
0.8. The RF algorithm, in specific, statistically outperformed all the
other traditional algorithms. Deep Learning baselines were slightly
more accurate but had a higher computation cost in the training
step. VGG16 was the best-evaluated model, followed by the simple
CNN and RF, with no statistical difference between the last two.
Results suggest that there is still scope for further improvement in
using the traditional algorithms.
O Computer on the Beach é um evento técnico-científico que visa reunir profissionais, pesquisadores e acadêmicos da área de Computação, a fim de discutir as tendências de pesquisa e mercado da computação em suas mais diversas áreas.